31.01.2022. ReHyb envisions a hybrid exoskeleton which assists users in supporting rehabilitation processes and for activities of daily living. The smart exoskeleton should understand the need of the user and then promote the movements most effective for the training for the “right amount”.
But every user has a different need, and even the same task may be performed differently by the same person. This makes tuning of the exoskeleton control to a specific user is a big challenge. Human movements are inherently variable due to the noise in the sensory-motor system and making the decision about whether a particular movement should be fostered or hindered is very difficult to conclude from simply observing the movements. Nevertheless, experts in movement disorders are capable of inferring abnormality in the movements and designing the most effective rehabilitation plans for the patients. How do they do it?
Biological movements are believed to be generated based on some kinds of optimality criteria such as energy efficiency, and stability of the body posture. Movement experts are likely to be detecting any irregularities in optimality criteria from learned experiences. In ReHyb, therefore, we have been studying how we can model the difference in the control policy of movements executed by patients using machine learning techniques. We have been collecting a variety of movements and motor control strategies adopted by patients to perform ADL tasks, and modelling them using a machine learning technique, called reinforcement learning. The results will be used to cluster irregular movements executed by patients and derive the most suitable support strategies through our hybrid exoskeleton.
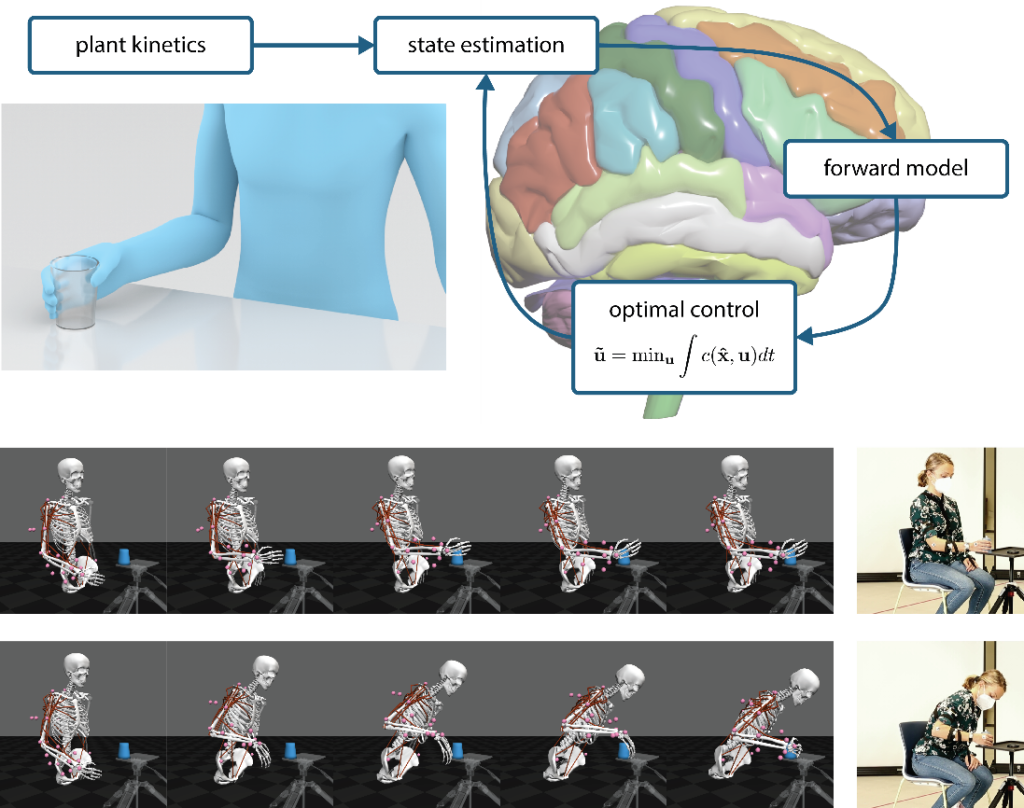